DSFSI @ ICLR 2023 - Kigali, Rwanda: 1-5 May 2023
We thrilled to have the opportunity to participate in and contribute to ICLR 2023 in Kigali
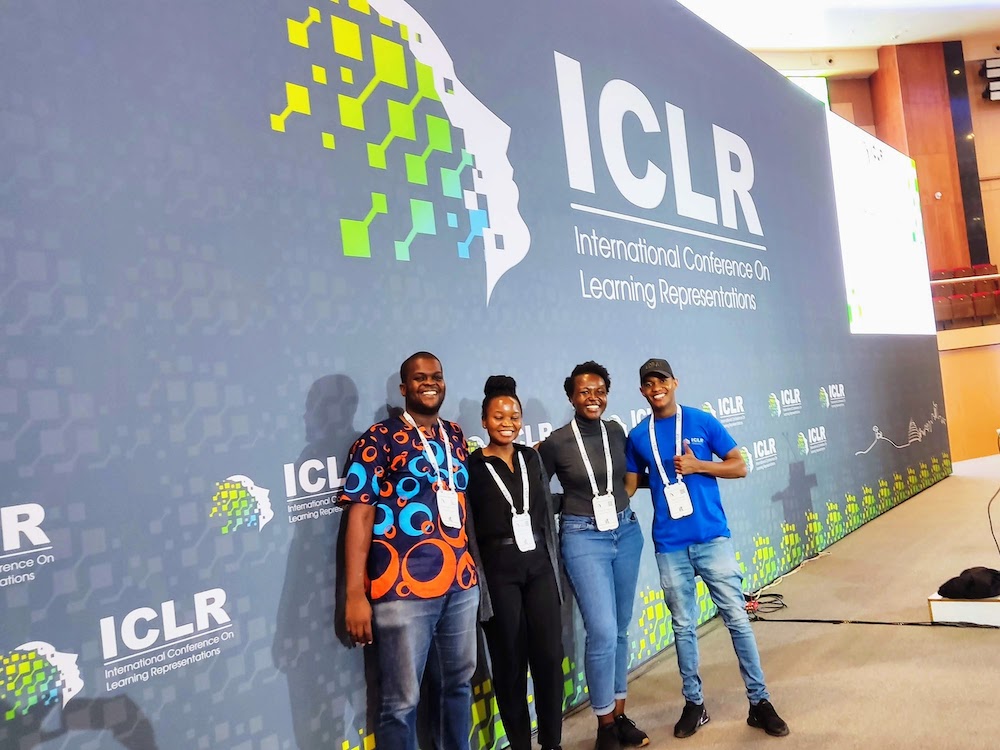
Data Science for Social Impact (DSFSI) is thrilled to have the opportunity to participate in and contribute to ICLR 2023, which marks the first time the International Conference on Learning Representations (ICLR) is being held on the African continent. We are especially proud that some of our members will be attending the conference in person in Kigali, Rwanda.
Member activities
Vukosi Marivate, PI @ DSFSI, is one of the program chairs for ICLR 2023 and has spent the last year working with the Program Chairs planning and organising the conference. Vukosi will give a keynote talk on Thursday at the IndabaX Rwanda Workshop.
Kathleen Siminyu, Masters Student @ DSFSI, will give two talks. The first titled ‘Reflections on Dataset Building, Ideas for Benefit Sharing’ at the IndabaX Rwanda Workshop and the second titled ‘Honouring Kiswahili with Technology and Community’ at the Practical Machine Learning for Developing Countries workshop. She will also take part in a panel titled ‘AI moving forward, leaving no one behind’ at the Women in Machine Learning Social.
Rozina Myoya, Masters Student @ DSFSI, will participate in the AfricaNLP workshop by showcasing the research carried out during the summer recess internship held by DSFSI. The study titled, “Fine-tuning Multilingual Pretrained African Language models”, explored the research question of whether PLMs can be fine-tuned to perform similarly well on different African language data. The ultimate goal is to increase the ability to effectively perform downstream NLP tasks such as text classification, name-entity-recognition and sentiment analysis, on African languages.
*Thapelo Sindane, Masters student @DSFSI research group will be doing volunteer work at the ICLR conference. He will be part of the volunteer team that assists speakers through talks, assists in coordinating track sessions, setup posters during poster sessions, and keeps track of ICLR website. In his free time he attends workshops, talks, and poster sessions for learning recent state-of-the art technologies in the AI space.
Papers @ AfricaNLP Workshop
MphayaNER: Named Entity Recognition for Tshivenda
Rendani Mbuvha, David I. Adelani, Tendani Mutavhatsindi, Tshimangadzo Rakhuhu, Aluwani Mauda, Tshifhiwa Joshua Maumela, Andisani Masindi, Seani Rananga, Vukosi Marivate, Tshilidizi Marwala
Preprint: https://arxiv.org/abs/2304.03952
Named Entity Recognition (NER) plays a vital role in various Natural Language Processing tasks such as information retrieval, text classification, and question answering. However, NER can be challenging, especially in low-resource languages with limited annotated datasets and tools. This paper adds to the effort of addressing these challenges by introducing MphayaNER, the first Tshivenda NER corpus in the news domain. We establish NER baselines by \textit{fine-tuning} state-of-the-art models on MphayaNER. The study also explores zero-shot transfer between Tshivenda and other related Bantu languages, with chiShona and Kiswahili showing the best results. Augmenting MphayaNER with chiShona data was also found to improve model performance significantly. Both MphayaNER and the baseline models are made publicly available.
FINE-TUNING MULTILINGUAL PRETRAINED AFRICAN LANGUAGE MODELS
Rozina Lucy Myoya, Fiskani Banda, Vukosi Marivate, Abiodun Modupe
Preprint: https://openreview.net/forum?id=5ybmJiXmIC
With the recent increase in low-resource African language text corpora , there have been advancements which have led to development of multilingual pre-trained language models (PLMs), based on African languages. These PLMS include AfriBerta, Afro-XLMR and AfroLM , which perform significantly well. The downstream tasks of these models range from text classification , name-entity-recognition and sentiment analysis. By exploring the idea of fine-tuning the different PLMs, these models can be trained on different African language datasets. This could lead to multilingual models that can perform well on the new data for the required downstream task of classification. This leads to the question we are attempting to answer: Can these PLMs be fine-tuned to perform similarly well on different African language data?