DSFSI @ Deep Learning IndabaX 2023 - Cape Town, South Africa: 12-14 July 2023
We thrilled to have the opportunity to participate in and contribute to Deep Learning IndabaX 2023 in Cape Town, South Africa
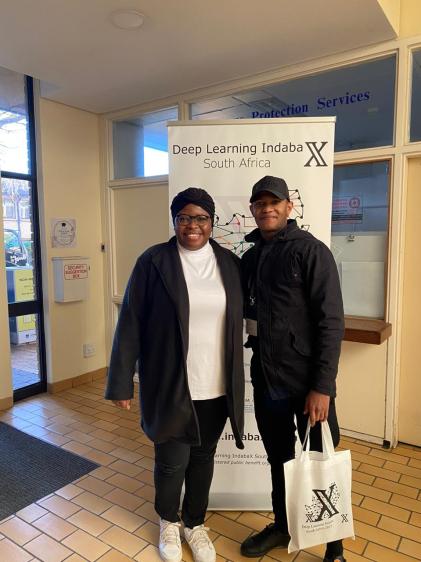
DSFSI (Data Science for Social Impact) is thrilled to have the opportunity to participate in and contribute to IndabaX South Africa 2023, being held in Cape Town 12-14 July 2023. We are especially proud that some of our members will be attending the conference in person.
Member activities
Thapelo Sindane, Masters student @DSFSI is an Organiser at the Deep Learning IndabaX, Cape Town, South Africa. His main role at the IndabaX included fundraising. Apart from being in the administrative committee, he presented a poster on “Zero-shot transfer learning using affix and correlated cross-lingual embeddings” . This work focuses on investigating novel alternative approaches to developing languages technologies for low-resourced languages such as transfer learning, conjoints embedding, e.t.c. This work is submitted for publication and is still under review.
Fiskani Banda, Masters Student @DSFSI, presented a poster based on a study titled “Fine-tuning Multilingual Pre-Trained African Language models” . This research was completed during the 2022 DSFSI summer recess internship. This research attempted to answer the research question : “Can these Pre-trained Language Models be fine-tuned to perform similarly well on different low resource languages (e.g., South African Language)?” To answer this question, an experiment was conducted, where pre- and post processing methods were investigated. The presentation of this work placed first overall for the poster session.
Papers
FINE-TUNING MULTILINGUAL PRETRAINED AFRICAN LANGUAGE MODELS
Rozina Lucy Myoya, Fiskani Banda, Vukosi Marivate, Abiodun Modupe
Preprint: https://openreview.net/forum?id=5ybmJiXmIC
With the recent increase in low-resource African language text corpora , there have been advancements which have led to development of multilingual pre-trained language models (PLMs), based on African languages. These PLMS include AfriBerta, Afro-XLMR and AfroLM , which perform significantly well. The downstream tasks of these models range from text classification , name-entity-recognition and sentiment analysis. By exploring the idea of fine-tuning the different PLMs, these models can be trained on different African language datasets. This could lead to multilingual models that can perform well on the new data for the required downstream task of classification. This leads to the question we are attempting to answer: Can these PLMs be fine-tuned to perform similarly well on different African language data?