UP<>Sweden AI Workshop Panel: Low Resource Languages and LLMs
UP<>Sweden AI Workshop Panel: Low Resource Languages and LLMs
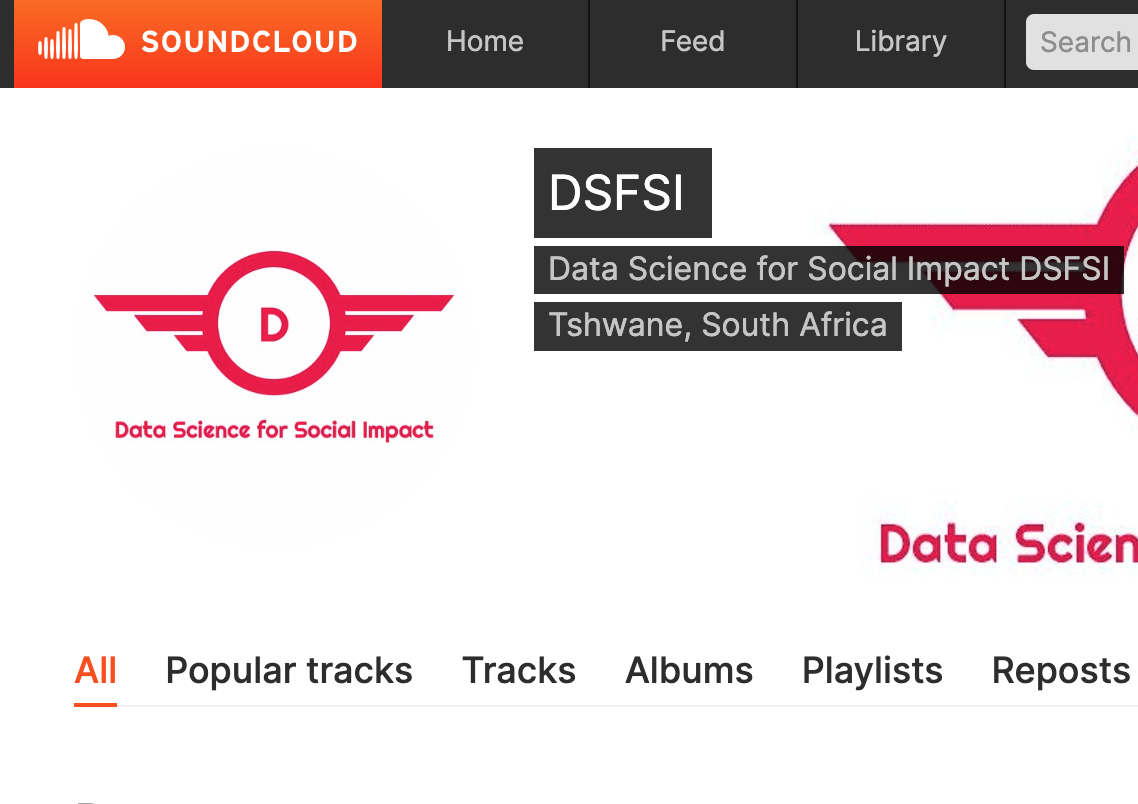
Listen
Summary
This panel discussion focused on the development of large language models (LLMs) for low-resource languages, particularly within the African context. The conversation emphasised the unique challenges of building AI systems for languages that have limited computational and linguistic resources. Here’s a summary of the main points:
Discussion was moderated by Lesego Makhafola from the University of Pretoria Library.
Introduction to Panellists and Their Work
- Nomonde Khalo: PhD student at the University of Cape Town, focusing on using AI and NLP to simplify medical texts for better comprehension. Her work highlights the need for personalised health communication.
- Vukosi Marivate: Expert in machine learning and NLP, he emphasised the lack of computational resources for many African languages and highlighted the need for collaborative, interdisciplinary approaches to address this gap.
- Chijioke Okorie: Legal expert with a focus on data science law, addressing issues around intellectual property and data rights in the context of AI and low-resource languages.
- Paul DeSantos: Data professional working on AI models for Nordic languages, contributed by discussing how low-resource languages could be addressed through transfer learning and data augmentation.
Key Themes and Challenges
-
Data Scarcity and Computational Resources:
- African languages are often underrepresented in NLP datasets, especially given the continent’s linguistic diversity (with over 3,000 languages). This lack of data, combined with limited access to high-performance computing, makes it difficult to build robust AI models.
- Building these models is not just a technical issue but also involves complex socio-cultural dynamics, including how languages are written, spoken, and understood.
-
Building AI with Community Input:
- The panel agreed that it is essential to develop AI models with input from local communities to ensure they are culturally relevant and free from bias. AI models should not be generic but rather tailored to specific tasks and community needs.
- The challenge is how to engage communities meaningfully, particularly in cases where languages are predominantly spoken rather than written.
-
Bias and Linguistic Representation:
- Models for low-resource languages are often biased due to the sources of the data, such as religious texts, which do not always represent the full linguistic or cultural context of the community. There was discussion about the importance of ensuring that datasets are diverse and culturally relevant.
- Panellists emphasised the importance of advocating for the use of local languages in business and online spaces to help grow these datasets and preserve linguistic diversity.
-
Legal Considerations:
- Dr. Okorie highlighted legal issues around data ownership and the ethical use of data, especially in light of privacy laws like South Africa’s Protection of Personal Information Act (POPIA). The legal framework should balance innovation with data protection, ensuring compliance while fostering openness.
- She also discussed the implications of data licensing, suggesting that data generated by public institutions should be openly shared to encourage innovation, while cautioning against inequitable uses of openly licensed data by those with greater resources.
-
Sustainability and Commercialization:
- There was a lively discussion on balancing the need for open access to data and models with the potential for commercialization. The panellists agreed that openness should not preclude value exchange, and that value doesn’t always have to be monetary—it could involve access to infrastructure, collaborative opportunities, or shared research benefits.
- Vukosi Marivate noted that it’s essential for open datasets and AI models developed in Africa to benefit local communities rather than being used by outside entities for profit without giving back.
Future Directions
- Transfer Learning and Data Augmentation: Paul DeSantos discussed current techniques such as transfer learning and data augmentation, which can be used to fine-tune models for low-resource languages. These approaches are data-efficient and allow for more targeted solutions.
- Crowdsourcing and Community-Driven Data Collection: The panel proposed that community-driven efforts, similar to initiatives like OpenStreetMap, could help build the necessary datasets for low-resource languages. This would democratise data collection and encourage local participation.
- Role of Universities and Libraries: Universities were identified as key players in bringing together disparate data resources. Libraries could also play a central role in managing language resources and making them accessible to researchers.
Conclusion
The panel concluded that addressing the challenges of developing AI for low-resource languages requires a multifaceted approach, combining technical innovation with community engagement, legal frameworks, and sustainable models for data sharing. Collaboration across disciplines—computer science, linguistics, law, and beyond—will be essential to ensure that AI systems reflect the linguistic diversity and cultural contexts of the regions they serve.